Registration Dossier
Registration Dossier
Data platform availability banner - registered substances factsheets
Please be aware that this old REACH registration data factsheet is no longer maintained; it remains frozen as of 19th May 2023.
The new ECHA CHEM database has been released by ECHA, and it now contains all REACH registration data. There are more details on the transition of ECHA's published data to ECHA CHEM here.
Diss Factsheets
Use of this information is subject to copyright laws and may require the permission of the owner of the information, as described in the ECHA Legal Notice.
EC number: 816-907-7 | CAS number: 1294454-93-5
- Life Cycle description
- Uses advised against
- Endpoint summary
- Appearance / physical state / colour
- Melting point / freezing point
- Boiling point
- Density
- Particle size distribution (Granulometry)
- Vapour pressure
- Partition coefficient
- Water solubility
- Solubility in organic solvents / fat solubility
- Surface tension
- Flash point
- Auto flammability
- Flammability
- Explosiveness
- Oxidising properties
- Oxidation reduction potential
- Stability in organic solvents and identity of relevant degradation products
- Storage stability and reactivity towards container material
- Stability: thermal, sunlight, metals
- pH
- Dissociation constant
- Viscosity
- Additional physico-chemical information
- Additional physico-chemical properties of nanomaterials
- Nanomaterial agglomeration / aggregation
- Nanomaterial crystalline phase
- Nanomaterial crystallite and grain size
- Nanomaterial aspect ratio / shape
- Nanomaterial specific surface area
- Nanomaterial Zeta potential
- Nanomaterial surface chemistry
- Nanomaterial dustiness
- Nanomaterial porosity
- Nanomaterial pour density
- Nanomaterial photocatalytic activity
- Nanomaterial radical formation potential
- Nanomaterial catalytic activity
- Endpoint summary
- Stability
- Biodegradation
- Bioaccumulation
- Transport and distribution
- Environmental data
- Additional information on environmental fate and behaviour
- Ecotoxicological Summary
- Aquatic toxicity
- Endpoint summary
- Short-term toxicity to fish
- Long-term toxicity to fish
- Short-term toxicity to aquatic invertebrates
- Long-term toxicity to aquatic invertebrates
- Toxicity to aquatic algae and cyanobacteria
- Toxicity to aquatic plants other than algae
- Toxicity to microorganisms
- Endocrine disrupter testing in aquatic vertebrates – in vivo
- Toxicity to other aquatic organisms
- Sediment toxicity
- Terrestrial toxicity
- Biological effects monitoring
- Biotransformation and kinetics
- Additional ecotoxological information
- Toxicological Summary
- Toxicokinetics, metabolism and distribution
- Acute Toxicity
- Irritation / corrosion
- Sensitisation
- Repeated dose toxicity
- Genetic toxicity
- Carcinogenicity
- Toxicity to reproduction
- Specific investigations
- Exposure related observations in humans
- Toxic effects on livestock and pets
- Additional toxicological data
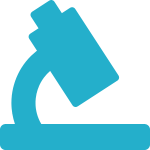
Partition coefficient
Administrative data
- Endpoint:
- partition coefficient
- Type of information:
- (Q)SAR
- Adequacy of study:
- key study
- Reliability:
- 2 (reliable with restrictions)
- Rationale for reliability incl. deficiencies:
- results derived from a valid (Q)SAR model and falling into its applicability domain, with adequate and reliable documentation / justification
- Justification for type of information:
- 1. SOFTWARE
EPI Suite™-Estimation Program Interface
2. MODEL (incl. version number)
KOWWIN™ v1.68
3. SMILES OR OTHER IDENTIFIERS USED AS INPUT FOR THE MODEL
Smiles code: O=C(C1CC1)N1CCN(CC1)Cc1ccccc1
4. SCIENTIFIC VALIDITY OF THE (Q)SAR MODEL
- Defined endpoint:
log Kow (log P) – logarithmic octanol/water partition coefficient. The partition coefficient Kow (P) is the ratio of concentrations of a chemical in n-octanol and in water at equilibrium at a specified temperature (typically 25 °C, although partition coefficient is not usually very temperature dependent and training data for KOWWIN are collected at different temperatures).
- Unambiguous algorithm:
KOWWIN uses a "fragment constant" methodology to predict log P. KOWWIN’s methodology is known as an Atom/Fragment Contribution (AFC) method. Coefficients for individual fragments and groups were derived by multiple regression of 2447 reliably measured log P values.
To estimate log P, KOWWIN initially separates a molecule into distinct atom/fragments. In general, each non-hydrogen atom in a structure is a "core" for a fragment; the exact fragment is determined by what is connected to the atom. Several functional groups are treated as core atoms. Connections to each core "atom" are either general or specific;specific connections take precedence over general connections.
Log P estimates made from atom/fragment values alone could or need to be improved by inclusion of substructures larger or more complex than "atoms"; hence, correction factors were added to the AFC method. They are either factors involving aromatic ring substituent positions, or miscellaneous factors. Correction factors are values for various steric interactions, hydrogen-bondings, and effects from polar functional substructures. Individual correction factors were selected through a tedious process of correlating the differences (between log P estimates from atom/fragments alone and measured log P values) with common substructures.
The general regression equation has the following form:
log P = ∑(f(i)*n(i)) + ∑(c(j)*n(j)) + b
where f(i) is the coefficient of atom/fragment i, n(i) – the number of times the fragment i occurs in the molecule, c(j) is the coefficient for the correction factor j, and n(j) the number of times the factor j occurs (or is applied) in the molecule. b is the linear equation constant; b = 0.229.
Values of f and c coefficients are available.
- Defined domain of applicability:
Currently there is no universally accepted definition of model domain. However, it should be considered that log P estimates may be less accurate for compounds outside the molecular weight range of the training set compounds, and/or that have more instances of a given fragment than the maximum for all training set compounds. Although the training set of the model contains a large number of diverse molecules and can be considered abundant, it is also possible that a compound may be characterised by structural features (e.g. functional groups) not represented in the training set, with no respective fragment/correction coefficient developed. These points should be taken into consideration when interpreting model results.
Training set molecular weights:
Minimum MW: 18.02
Maximum MW: 719.82 (in the validation set: 991.15)
Average in the training set: 199.98.
- Appropriate measures of goodness-of-fit and robustness and predictivity:
Training set statistics:
N = 2447 compounds
correlation coefficient R2= 0.982
standard deviation = 0.217
absolute deviation = 0.159
Training set estimation error:
within ≤ 0.10 – 45.0%
within ≤ 0.20 – 72.5%
within ≤ 0.40 – 92.4%
within ≤ 0.50 – 96.4%
within ≤ 0.60 – 98.2%
External validation set statistics:
N = 10946 compounds
correlation coefficient R2= 0.943
standard deviation = 0.479
absolute deviation = 0.356
Validation set estimation error:
within ≤ 0.20 – 39.6%
within ≤ 0.40 – 66.0%
within ≤ 0.50 – 75.6%
within ≤ 0.60 – 82.5%
within ≤ 0.80 – 91.6%
within ≤ 1.00 – 95.6%
- Mechanistic interpretation:
KOWWIN’s "reductionist" fragment constant methodology (i.e. derivation via multiple regression) differs from the "constructionist" fragment constant methodology of Hansch and Leo (Hansch, C. and Leo, A.J., Substituent Constants for Correlation Analysis in Chemistry and Biology, Wiley, New York, 1979). More complete description of KOWWIN methodology is described in: Meylan, W.M., and Howard, P.H., Atom/Fragment Contribution Method for Estimating Octanol-Water Partition Coefficients, J. Pharm. Sci 84: 83-92, 1995.
5. APPLICABILITY DOMAIN
- Descriptor domain:
The substance has a molecular weight of 244.34 and is therefore in the molecular weight range of the compounds in the training set (between 18 and 720)
- Structural and mechanistic domains:
The number of each fragment found in the substance does not exceed the maximum number of this fragment that occurs in any individual compound of the training set.
- Similarity with analogues in the training set:
The prediction for a similar substance 1,4-Benzodiazpin-2-one,5(2FPh)7CL (CAS 75349-23-4) results in a logKow of 0.31. The experimental result for this substance indicates a logKow of 0.35 (Hansch,C & Leo,A, 1985). This supports the use of the model for the substance.
6. ADEQUACY OF THE RESULT
The substance fits in the applicability domain of the model. The prediction is valid and can be used for classification and risk assessment.
Data source
Reference
- Reference Type:
- study report
- Title:
- Unnamed
- Year:
- 2 018
- Report date:
- 2018
Materials and methods
- Principles of method if other than guideline:
- - Justification of QSAR prediction: see field 'Justification for type of information'
- GLP compliance:
- no
- Type of method:
- calculation method (fragments)
- Partition coefficient type:
- octanol-water
Test material
- Reference substance name:
- 1-benzyl-4-(cyclopropylcarbonyl)piperazin-1-ium chloride
- EC Number:
- 816-907-7
- Cas Number:
- 1294454-93-5
- Molecular formula:
- C15H20N2O.ClH
- IUPAC Name:
- 1-benzyl-4-(cyclopropylcarbonyl)piperazin-1-ium chloride
Constituent 1
Results and discussion
Partition coefficient
- Type:
- log Pow
- Partition coefficient:
- 1.6
- Remarks on result:
- other: QSAR
Applicant's summary and conclusion
Information on Registered Substances comes from registration dossiers which have been assigned a registration number. The assignment of a registration number does however not guarantee that the information in the dossier is correct or that the dossier is compliant with Regulation (EC) No 1907/2006 (the REACH Regulation). This information has not been reviewed or verified by the Agency or any other authority. The content is subject to change without prior notice.
Reproduction or further distribution of this information may be subject to copyright protection. Use of the information without obtaining the permission from the owner(s) of the respective information might violate the rights of the owner.
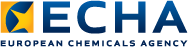